Using BGLS with MPS to solve QAOA problems#
For large sparse graphs, we expect matrix product states to be particularly efficient being that their runtime scales primarily in the (sparse) degree of connectedness. Here we will use such states with our bgls sampler to solve the MaxCut problem for random graphs.
import time
import numpy as np
import matplotlib.pyplot as plt
import sympy
import networkx as nx
import cirq
import cirq.contrib.quimb as ccq
import cirq.contrib.quimb.mps_simulator
from cirq.contrib.svg import SVGCircuit
import quimb.tensor as qtn
import bgls
Here we define a function to compute bitstring amplitudes for a matrix product state. (Note, this is the same as found in mps_simulation)
def cirq_mps_bitstring_probability(
mps: cirq.contrib.quimb.MPSState, bitstring: str
) -> float:
"""
Returns the probability of measuring the `bitstring` (|z⟩) in the
'cirq.contrib.quimb.MPSState' mps.
Args:
mps: Matrix Product State as a 'cirq.contrib.quimb.MPSState'.
bitstring: Bitstring |z⟩ as a binary string.
"""
M_subset = []
for i, Ai in enumerate(mps.M):
qubit_index = mps.i_str(i)
# selecting the component with matching bitstring:
A_subset = Ai.isel({qubit_index: int(bitstring[i])})
M_subset.append(A_subset)
tensor_network = qtn.TensorNetwork(M_subset)
bitstring_amplitude = tensor_network.contract(inplace=False)
return np.power(np.abs(bitstring_amplitude), 2)
We first create a random graph with n nodes and connectivity p
n = 10
p = 0.3
rand_graph = nx.erdos_renyi_graph(n, p, seed=2)
nx.draw(rand_graph, with_labels=True)
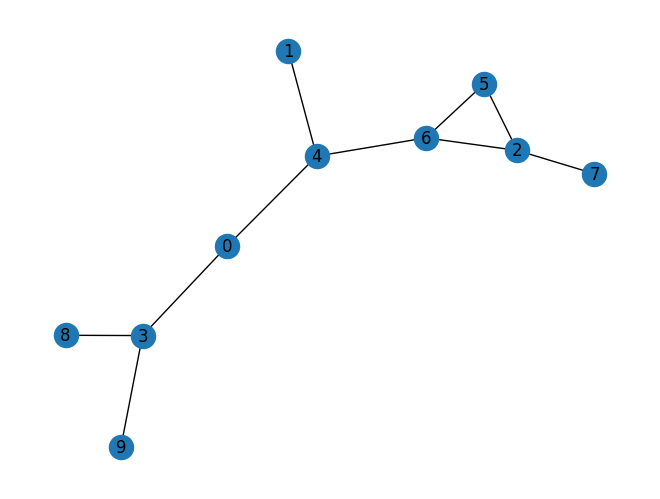
We then implement the cost hamiltonian translated to qubits \(H_c = \frac{1}{2}\sum(1-Z_iZ_j)\), parametrized as \(U_c=e^{i\gamma H_c}\), as well as the mixing hamiltonian \(H_m = \sum X_i\), parametrized as \(U_m=e^{i\beta H_m}\).
def config_energy(assignments, graph):
energy = 0.
for (i, j) in graph.edges:
energy += 1 - (assignments[i] * assignments[j])
energy /= 2
return energy
def obj_func(result, graph):
# return the average energy for a results repetitions
def config_energy(assignments):
energy = 0.
for (i, j) in graph.edges:
energy += 1 - (assignments[i] * assignments[j])
energy /= 2
return energy
assignments = 2 * result.measurements.get("z") - 1 # convert to +- 1
return np.average(
[config_energy(assignment) for assignment in assignments])
def Uc(graph, gamma):
for (i, j) in graph.edges:
yield cirq.ZZ.on(cirq.LineQubit(i), cirq.LineQubit(j)) ** gamma
def Um(graph, beta):
for i in graph.nodes:
yield cirq.X.on(cirq.LineQubit(i)) ** beta
def construct_circuit(graph, p_range, gammas, betas):
circuit = cirq.Circuit()
for i in graph.nodes:
circuit.append(cirq.H.on(cirq.LineQubit(i)))
for p in range(p_range):
circuit.append(Uc(graph, gammas[p]))
circuit.append(Um(graph, betas[p]))
circuit.append(
cirq.measure(cirq.LineQubit.range(len(graph.nodes)), key='z'))
return circuit
g1 = sympy.Symbol("g1")
b1 = sympy.Symbol("b1")
maxcut_circuit = construct_circuit(rand_graph, 1, [g1], [b1])
SVGCircuit(maxcut_circuit)
findfont: Font family 'Arial' not found.
findfont: Font family 'Arial' not found.
findfont: Font family 'Arial' not found.
findfont: Font family 'Arial' not found.
findfont: Font family 'Arial' not found.
findfont: Font family 'Arial' not found.
findfont: Font family 'Arial' not found.
findfont: Font family 'Arial' not found.
findfont: Font family 'Arial' not found.
findfont: Font family 'Arial' not found.
findfont: Font family 'Arial' not found.
findfont: Font family 'Arial' not found.
findfont: Font family 'Arial' not found.
findfont: Font family 'Arial' not found.
findfont: Font family 'Arial' not found.
findfont: Font family 'Arial' not found.
findfont: Font family 'Arial' not found.
findfont: Font family 'Arial' not found.
findfont: Font family 'Arial' not found.
findfont: Font family 'Arial' not found.
findfont: Font family 'Arial' not found.
findfont: Font family 'Arial' not found.
findfont: Font family 'Arial' not found.
findfont: Font family 'Arial' not found.
findfont: Font family 'Arial' not found.
findfont: Font family 'Arial' not found.
findfont: Font family 'Arial' not found.
findfont: Font family 'Arial' not found.
findfont: Font family 'Arial' not found.
findfont: Font family 'Arial' not found.
findfont: Font family 'Arial' not found.
findfont: Font family 'Arial' not found.
findfont: Font family 'Arial' not found.
findfont: Font family 'Arial' not found.
findfont: Font family 'Arial' not found.
findfont: Font family 'Arial' not found.
findfont: Font family 'Arial' not found.
findfont: Font family 'Arial' not found.
findfont: Font family 'Arial' not found.
findfont: Font family 'Arial' not found.
findfont: Font family 'Arial' not found.
findfont: Font family 'Arial' not found.
findfont: Font family 'Arial' not found.
findfont: Font family 'Arial' not found.
findfont: Font family 'Arial' not found.
findfont: Font family 'Arial' not found.
findfont: Font family 'Arial' not found.
findfont: Font family 'Arial' not found.
findfont: Font family 'Arial' not found.
findfont: Font family 'Arial' not found.
findfont: Font family 'Arial' not found.
findfont: Font family 'Arial' not found.
findfont: Font family 'Arial' not found.
findfont: Font family 'Arial' not found.
findfont: Font family 'Arial' not found.
findfont: Font family 'Arial' not found.
findfont: Font family 'Arial' not found.
findfont: Font family 'Arial' not found.
findfont: Font family 'Arial' not found.
findfont: Font family 'Arial' not found.
We find the optimal values of gamma and beta by sweeping across parameter combinations. Sampling repeatedly from the resultant circuits, and computing the final average energy for each, we pick the combination minimizing the energy. We can make use of symbols to call cirq’s run_sweep
across a range of settings.
ngammas = 8
nbetas = 8
param_sweep = (cirq.Linspace(key='g1', start=0.1, stop=0.9,
length=ngammas) * cirq.Linspace(key='b1',
start=0.1,
stop=0.9,
length=nbetas))
class LowChi(cirq.contrib.quimb.MPSOptions):
max_bond = 3
bgls_mps_sampler = bgls.Simulator(
cirq.contrib.quimb.MPSState(
qubits=maxcut_circuit.all_qubits(), initial_state=0,
prng=np.random.RandomState(), simulation_options=LowChi()
),
cirq.act_on,
cirq_mps_bitstring_probability,
seed=1
)
results = bgls_mps_sampler.run_sweep(maxcut_circuit, params=param_sweep,
repetitions=100)
---------------------------------------------------------------------------
KeyboardInterrupt Traceback (most recent call last)
Cell In[7], line 1
----> 1 results = bgls_mps_sampler.run_sweep(maxcut_circuit, params=param_sweep,
2 repetitions=100)
File /opt/hostedtoolcache/Python/3.11.5/x64/lib/python3.11/site-packages/cirq/sim/simulator.py:72, in SimulatesSamples.run_sweep(self, program, params, repetitions)
69 def run_sweep(
70 self, program: 'cirq.AbstractCircuit', params: 'cirq.Sweepable', repetitions: int = 1
71 ) -> Sequence['cirq.Result']:
---> 72 return list(self.run_sweep_iter(program, params, repetitions))
File /opt/hostedtoolcache/Python/3.11.5/x64/lib/python3.11/site-packages/cirq/sim/simulator.py:103, in SimulatesSamples.run_sweep_iter(self, program, params, repetitions)
101 records[protocols.measurement_key_name(op)] = np.empty([0, 1, 1])
102 else:
--> 103 records = self._run(
104 circuit=program, param_resolver=param_resolver, repetitions=repetitions
105 )
106 yield study.ResultDict(params=param_resolver, records=records)
File /opt/hostedtoolcache/Python/3.11.5/x64/lib/python3.11/site-packages/bgls/simulator.py:125, in Simulator._run(self, circuit, param_resolver, repetitions)
122 param_resolver = param_resolver or cirq.ParamResolver()
123 resolved_circuit = cirq.resolve_parameters(circuit, param_resolver)
--> 125 return self._sample(resolved_circuit, repetitions)
File /opt/hostedtoolcache/Python/3.11.5/x64/lib/python3.11/site-packages/bgls/simulator.py:148, in Simulator._sample(self, circuit, repetitions)
143 keys_to_bitstrings_list = []
145 if not needs_trajectories(circuit):
146 # Sample all bitstrings in one pass through the circuit.
147 keys_to_bitstrings_list = (
--> 148 self._sample_from_one_wavefunction_evolution(
149 circuit, repetitions
150 )
151 )
152 else:
153 # Sample one bitstring per trajectory.
154 for _ in range(repetitions):
File /opt/hostedtoolcache/Python/3.11.5/x64/lib/python3.11/site-packages/bgls/simulator.py:219, in Simulator._sample_from_one_wavefunction_evolution(self, circuit, repetitions)
215 self._apply_op(op, state)
217 # Skip updating bitstrings for diagonal gates since they do not change
218 # the probability distribution.
--> 219 if all(cirq.is_diagonal(kraus) for kraus in cirq.kraus(op)):
220 continue
222 # Memoize self._compute_probability.
File /opt/hostedtoolcache/Python/3.11.5/x64/lib/python3.11/site-packages/cirq/protocols/kraus_protocol.py:154, in kraus(val, default)
151 return tuple(np.sqrt(p) * u for p, u in mixture_result)
153 unitary_getter = getattr(val, '_unitary_', None)
--> 154 unitary_result = NotImplemented if unitary_getter is None else unitary_getter()
155 if unitary_result is not NotImplemented and unitary_result is not None:
156 return (unitary_result,)
File /opt/hostedtoolcache/Python/3.11.5/x64/lib/python3.11/site-packages/cirq/ops/gate_operation.py:195, in GateOperation._unitary_(self)
193 getter = getattr(self.gate, '_unitary_', None)
194 if getter is not None:
--> 195 return getter()
196 return NotImplemented
File /opt/hostedtoolcache/Python/3.11.5/x64/lib/python3.11/site-packages/cirq/ops/eigen_gate.py:342, in EigenGate._unitary_(self)
340 return NotImplemented
341 e = cast(float, self._exponent)
--> 342 return np.sum(
343 [
344 component * 1j ** (2 * e * (half_turns + self._global_shift))
345 for half_turns, component in self._eigen_components()
346 ],
347 axis=0,
348 )
File /opt/hostedtoolcache/Python/3.11.5/x64/lib/python3.11/site-packages/numpy/core/fromnumeric.py:2313, in sum(a, axis, dtype, out, keepdims, initial, where)
2310 return out
2311 return res
-> 2313 return _wrapreduction(a, np.add, 'sum', axis, dtype, out, keepdims=keepdims,
2314 initial=initial, where=where)
File /opt/hostedtoolcache/Python/3.11.5/x64/lib/python3.11/site-packages/numpy/core/fromnumeric.py:88, in _wrapreduction(obj, ufunc, method, axis, dtype, out, **kwargs)
85 else:
86 return reduction(axis=axis, out=out, **passkwargs)
---> 88 return ufunc.reduce(obj, axis, dtype, out, **passkwargs)
KeyboardInterrupt:
We then find the combo with minimum energy by searching over all pairs
energies = np.zeros(shape=(ngammas, nbetas))
max_energy = None
max_params = None
for i, result in enumerate(results):
energy = obj_func(result, rand_graph)
energies[int(i / ngammas), i % nbetas] = energy
if max_energy == None or energy > max_energy:
max_energy = energy
max_params = result.params
plt.pcolormesh(np.linspace(0.1, 0.9, ngammas), np.linspace(0.1, 0.9, nbetas),
energies, shading="nearest")
plt.colorbar()
plt.xlabel(r"$\beta$")
plt.ylabel(r"$\gamma$");
plt.title("energy")
Text(0.5, 1.0, 'energy')
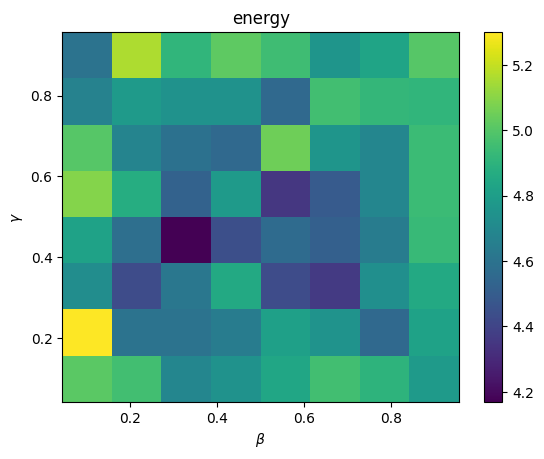
print("max energy: ", max_energy)
print("at params: ", max_params)
max energy: 5.3
at params: cirq.ParamResolver({'g1': 0.2142857142857143, 'b1': 0.1})
Finally we fix the maximized parameters, repeatedly sample at this configuration, and then take as our graph solution the measurement with maximal energy.
result_at_max = bgls_mps_sampler.run_sweep(maxcut_circuit, params=max_params,
repetitions=1000)
best_energies = np.zeros(shape=(ngammas, nbetas))
max_best_energy = None
best_assignment = None
for i, result in enumerate(result_at_max):
for assignment in 2 * result.measurements.get("z") - 1:
energy = config_energy(assignment, rand_graph)
best_energies[int(i / ngammas), i % nbetas] = energy
if max_best_energy == None or energy > max_best_energy:
max_best_energy = energy
best_assignment = assignment
print("max best energy i.e. number of slices: ", max_best_energy)
print("with system configuration: ", best_assignment)
max best energy i.e. number of slices: 9.0
with system configuration: [-1. -1. 1. 1. 1. -1. -1. -1. -1. -1.]
nodesa = [0, 1, 2, 6, 8, 9]
nodesb = [3, 4, 5, 7]
subset_color = ["red", "green"]
g = nx.Graph()
for n in rand_graph.nodes():
if n in nodesa:
g.add_node(node_for_adding=n, attr={'l': 0})
else:
g.add_node(node_for_adding=n, attr={'l': 1})
for a, b in rand_graph.edges:
g.add_edge(a, b)
colors = [subset_color[data['attr']['l']] for v, data in g.nodes(data=True)]
nx.draw(g, node_color=colors, with_labels=True)
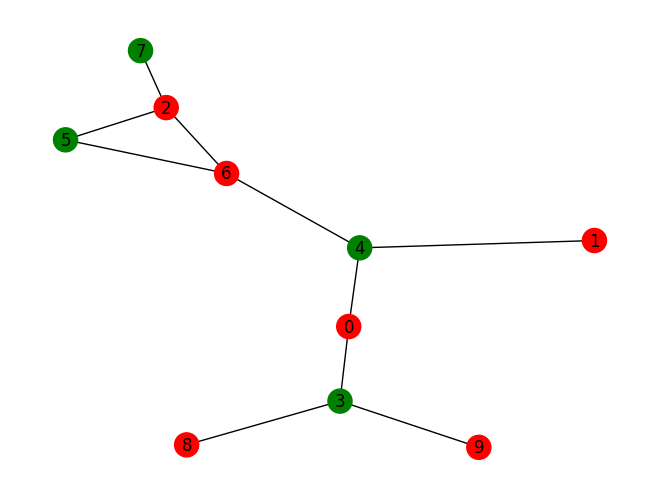